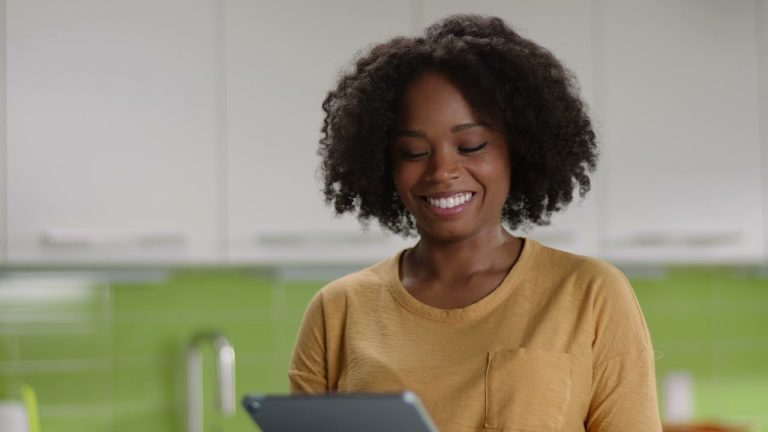
Harmful content evolves very quickly, so AI systems must keep up with it. To do this, the AI must learn what it is looking for, taking months to collect and label the thousands, if not millions, of examples needed to train each AI system to spot a new type of content. To address this, Meta AI has built and deployed a new AI technology called Few-Shot Learner (FSL) that is capable of adapting within weeks and taking action on new harmful content.
This AI system introduced by Meta AI works in over 100 languages and also learns from different types of data, such as images and text, and can augment existing AI models already deployed to detect other types of harmful content. It uses a relatively new method, called Few-Shot Learner, in which models start with a general understanding of many different topics and then use far fewer, and in some cases no, labeled examples to learn new tasks. If traditional systems are analogous to a fishing line capable of catching a specific type of fish, the SLF is an additional net capable of catching other types of fish as well.
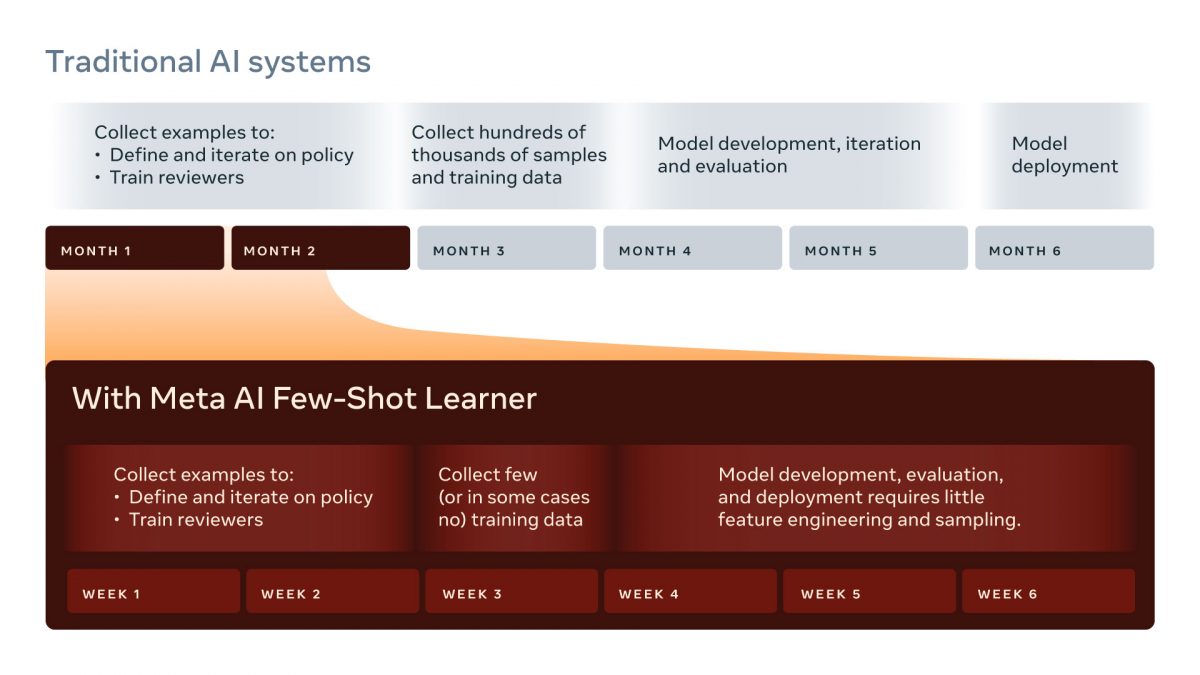
A system that would outperform traditional AI
The self-supervised learning techniques and the new infrastructure developed by Meta AI make it possible to go beyond traditional AI systems and move towards larger, more consolidated and generalized systems, less dependent on labeled data. Unlike previous systems that relied on matching models to labeled data, the FSL system is first trained on billions of examples of generic and free languages, then with labeled illegal content over time. Finally, it is trained on a condensed text explaining a new policy, so that it can learn the policy text implicitly.
The LSP was tested on novel events such as identifying content that shares misleading or sensational information that may discourage COVID-19 vaccinations (e.g., “Vaccine or DNA modifier?”). In another separate task, the new AI system enhanced an existing classifier that flags content close to inciting violence (e.g., “Does this guy need all his teeth?”). The traditional approach might have missed these types of messages because there aren’t many labeled examples that use DNA language to create vaccine hesitation or that refer to teeth to imply violence.
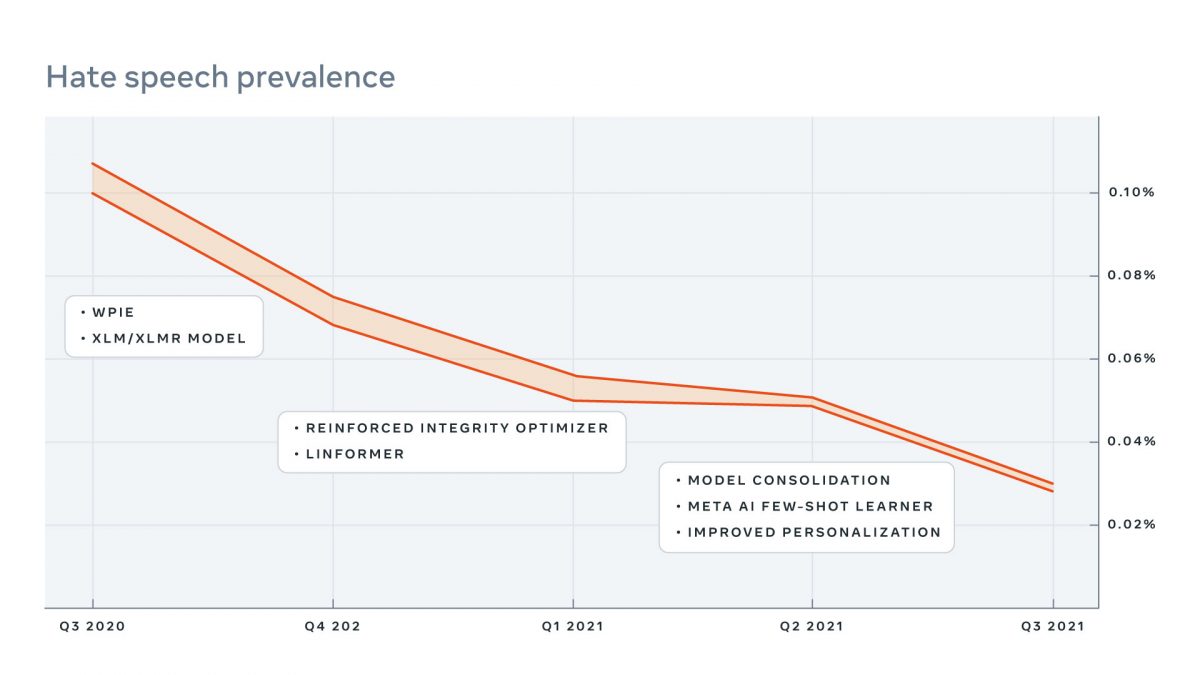
Meta AI conducted standardized offline and online A/B testing protocols to measure the performance of the model. In these tests, the delta between the prevalence of harmful content – that is, the percentage of views of infringing content that people see – before and after the LSP was deployed on Facebook and Instagram was examined. Meta AI Few-shot Learner was able to correctly detect posts that traditional systems can miss and thus helped reduce the prevalence of these types of harmful content. It does this by proactively detecting potentially harmful content and preventing it from spreading across platforms. It has also been found that, in combination with existing classifiers, the SLF has helped to reduce the prevalence of other harmful content, such as hate speech.
Significant progress towards a model that is touted as high performance
Meta AI teams are working on additional tests to improve classifiers that could benefit from more labeled training data, such as those from countries that speak languages without large volumes of labeled training data. These generalized intelligent AI models are still in their infancy. There is still a long way to go before AI can understand dozens of pages of policy text and know immediately and exactly how to apply it.
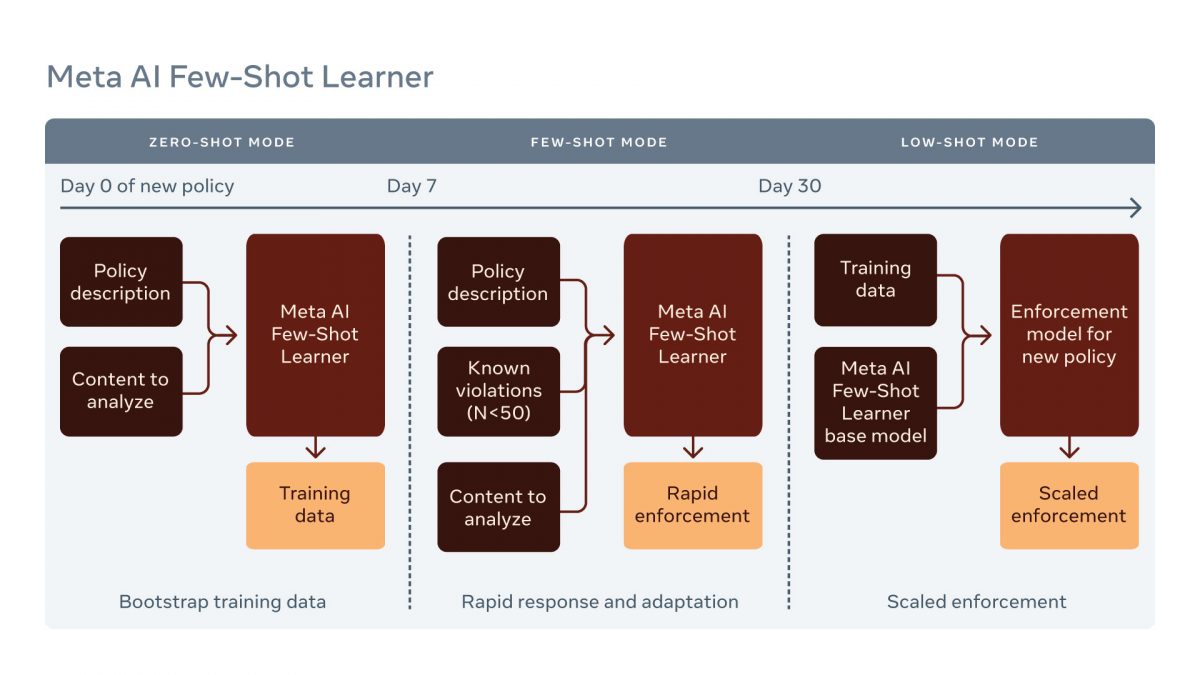
Few-Shot Learner is a large-scale, multi-modal, multi-lingual model that allows for joint understanding of policy and content, integrity issues and does not require fine-tuning of the model.
This new system operates in three different scenarios, each requiring varying levels of labeled examples:
- Zero-shot: Policy descriptions without examples.
- Low-shot with demonstration: Policy descriptions with a small set of examples (less than 50).
- Low-shot with fine-tuning: ML developers can fine-tune the basic FSL model with a small number of training examples.
The global input to FSL is composed of three parts. First, building on previous work with Whole Post Integrity Embeddings (WPIE), it learns multimodal information from the entire message, including text, image, URL, etc. Second, it analyzes policy-related information, such as the policy definition, or labeled examples that indicate whether or not a particular message violates the policy definition. Third, Meta AI designers also take additional labeled examples as demonstrations, if available.
In this new approach, called Entailment Few-Shot Learning, the key idea is to convert the class label into a natural language sentence that can be used to describe the label, and determine whether the example implies the label description. For example, a sentiment classification input and label pair can be rephrased as:
- [x: “I love your ethnic group. JK. You should all be six feet underground” y: positive] as following textual entailment sample:
- [x: I love your ethnic group. JK. You should all be 6 feet underground. This is hate speech. y: entailment].
Through a series of systematic evaluations, this method has been shown to outperform other few-shot learning methods by up to 55% (and 12% on average).
The Meta AI teams believe that SLF can, over time, improve the integrity performance of all their AI systems by allowing them to leverage a unique, shared knowledge base to handle many different types of violations. But it could also help policy, tagging and investigation workflows bridge the gap between human insight and classifier progress.
The hunt for illegal content is getting faster
The LSP can be used to test a new set of likely policy violations and understand the sensitivity and validity of proposed definitions. It casts a wider net, bringing to light more types of “near” content violations that policy teams need to be aware of when making a decision or formulating advice for annotators training new classifiers.
The ability to quickly begin enforcing policies against content types that do not have a large amount of labeled learning data is a major advance and will help make these systems more agile and responsive to new challenges. Few-shot and zero-shot learning is one of the many cutting-edge areas of AI in which Meta AI has made significant research investments. The teams are actively working on key open search problems that go beyond understanding content alone and attempt to infer the cultural, behavioral, and conversational context surrounding it.
Much work remains to be done, but these initial results are an important step that marks a move towards more intelligent and generalized AI systems that can quickly learn multiple tasks at once.
Meta AI’s long-term vision is to achieve human-like flexibility and learning efficiency, which will make their integrity systems even faster and easier to train and more capable of working with new information. An AI system such as Few-Shot Learner can significantly improve the social networking giant’s ability to detect and adapt to emerging situations. By identifying evolving and harmful content much more quickly and accurately, FSL promises to be a key piece of technology that will help Meta AI continue to evolve and address harmful content on its platforms.
Translated from Meta AI dévoile une technologie d’IA pour améliorer la modération sur Facebook et Instagram