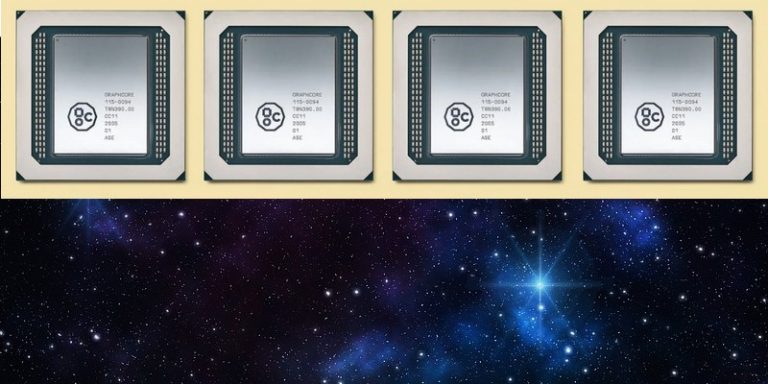
Artificial intelligence is increasingly used in astrophysics: recently, several Japanese researchers have exploited deep learningto better observe and understand the universe. Still in the field of cosmology, the University of Paris has taken advantage of Graphcore’s Intelligence Processing Unit (IPU) to accelerate research in the field. The neural networks used could be trained more quickly.
The problem of increasingly large and complex simulation data
In a study published at the beginning of June, researcher Bastien Arcelin describes two case studies involving deep learning: the creation of an image of a galaxy and the estimation of the shape of a galaxy. These and future research projects produce a significant amount of data from observations. To give an idea of what this might look like, the Vera C. Rubin Observatory’s Legacy Survey of Space and Time (LSST) project is expected to generate 20 terabytes (1012) of data each night, or about 60 petabytes (1015) after ten years.
In order to process this data, cosmology researchers are turning to neural networks to better manage these increasingly large data sets. Because simulation data are also complex and numerous, the associated neural network must be fast, accurate, and in some cases, capable of accurately characterizing epistemic uncertainty.
A problem has emerged: does equipment designed specifically for AI have superior performance to standard equipment for deep learning in cosmology? For the purposes of the study, the performance of a first generation Graphcore chip (the GC2 IPU) was compared to that of the Nvidia V100 GPU processor.
Estimation of galaxy shape parameters using neural networks
Bastien Arcelin, a researcher at the University of Paris, has developed a new technique involving deep neural networks and convolutional layers capable of measuring the ellipticity parameters of isolated and overlapping galaxies. He then tested his technique with a deterministic neural network and on a Bayesian network (a probabilistic graphical model):
- For neural networks: once trained, the parameters of deterministic networks are associated with a fixed value and do not change, even if the same image of an isolated galaxy is added twice to the network.
- For Bayesian networks: weights are associated with probability distributions, not with single values. Therefore, if you add the same image to the network twice, you get two different samples of probability distributions, and thus two slightly different results.
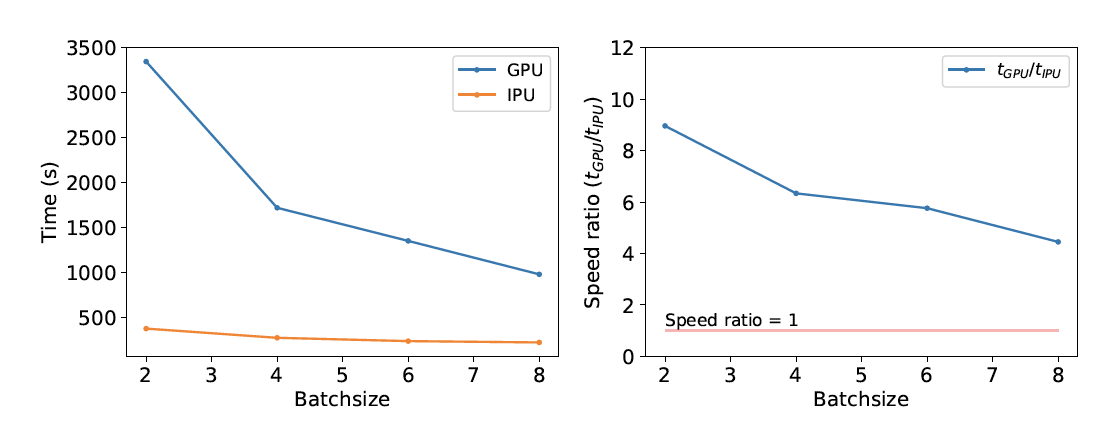
Graphcore IPUs exploited in future cosmological studies
When training small batches, the IPU was found to be twice as fast as the GPU with deep networks, and at least 4 times faster with Bayesian networks. The GC2 IPU was able to achieve such performance while consuming half the power of the GPU.
According to Graphcore, this study showed that the IPU technology can dramatically speed up the training phase for galaxy shape parameter estimates, and that an IPU offers better results with small batch sizes. For larger batches, multiple IPUs can be used to improve performance.
Translated from L’Université de Paris cherche à accélérer la recherche en cosmologie grâce aux IPU et aux réseaux de neurones