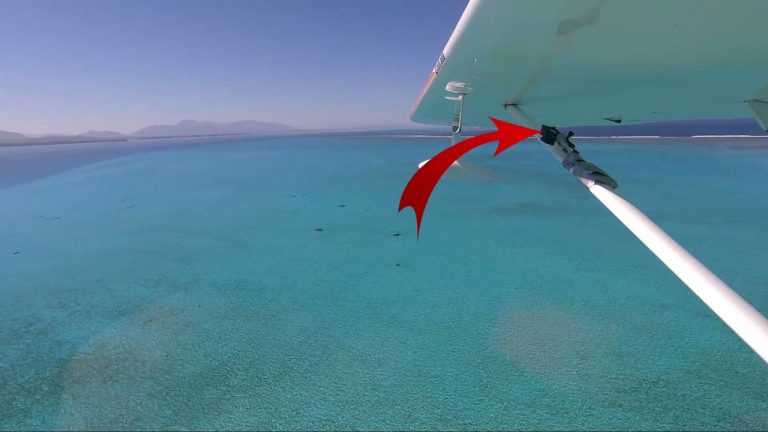
New Caledonia’s underwater fauna is very varied, but it is its major representatives that have caught Laura Manocci’s attention. To better protect these sometimes rare animals such as the dugong as well as various sharks, rays and turtles, the biologist from Marbec and her colleagues have imagined an aerial census… by microlight! Researchers from the Entropie, Limm and Marbec laboratories have collaborated on this innovative method integrating artificial intelligence and more precisely Deep Learning.
Until now, when we wanted to count the underwater fauna, we dove and brought back videos. Laura Manocci preferred to take to the skies and filmed the New Caledonian lagoon from a height of 50 to 60 metres aboard her microlight. She explained:
“These waters are very shallow, 2 metres maximum, so you can see the marine animals even at this altitude.”
The problem was then to identify the different animals that were moving in these images. Some are very fast, others rare like the dugong since there are no more than a thousand of them in New Caledonian waters. The researchers therefore used artificial intelligence:
“We resorted to what is called deep learning, artificial intelligence applied to pattern recognition.”
But to apply deep learning to this kind of algorithm, you need to be able to provide it with a large amount of images. The researchers went looking for them where there is a plethora and moreover free: social networks. Laura Manocci explained:
“The dugong is such an iconic animal that we figured that when people had the chance to film it, they would probably post the footage on social networks.”
For the dugong, they found 20 videos that they used to train a deep learning model, this program is able to detect and identify 80% of the animals on the videos.
“This method thus offers a new and powerful way to count and map dugongs and other charismatic marine species in order to better protect them. These censuses could lead to recommendations to place certain areas in strict reserves if necessary.
Making such algorithms remains a challenge, explains Laura Manocci:
“Building training databases is even more difficult for rare and endangered marine megafauna because most wild individuals remain in particular and often remote locations.”
They use an architecture of convolutional neural networks. These CNNs are deep learning algorithms widely used for image classification and object detection (simultaneously locating and classifying objects in images). They are often used to detect animals in images, identify and classify them, as was the case for the census of the dugong and other species in the New Caledonian lagoon.
FIGURE 1
Steps of the deep learning method that detects rare megafauna (CNN, convolutional neural network; TP, true positive; FP, false positive; FN, false negative). The sox network images (grey) are used in steps 2 and 3. Field images (black) are used in steps 2, 4 and 5.
- In step 1, social media videos of species of interest are collected by searching social media websites with appropriate keywords. In parallel, and independently, field video surveys are conducted in the study area.
- In step 2, images from the social media videos are extracted and annotated (bounding boxes are manually drawn around the species of interest). The annotated images are then partitioned into independent training and test sets, and the training set is artificially augmented. The field video survey images are also extracted and annotated.
- In step 3, a pre-trained and publicly available object detection CNN is downloaded and recycled for species detection on the social media dataset.
- In step 4, a CNN is applied to predict species detections on the field survey images.
- In step 5, the predicted detections are compared to the manually annotated boundary frames on the field images and the accuracy of the CNN is evaluated. Performance measures are calculated based on the number of true positives (TPs), false positives (FPs), and false negatives (FNs). A TP corresponds to an overlap between a predicted and an annotated box. A predicted bounding box that does not match an annotated bounding box is a TP, while an annotated bounding box that does not match a predicted bounding box is a FN. Accuracy is the percentage of TPs to predictions (equation 1). It represents the percentage of correct predictions (closest to 1, least FP).
Translated from L’intelligence artificielle au secours de la faune sous-marine calédonienne