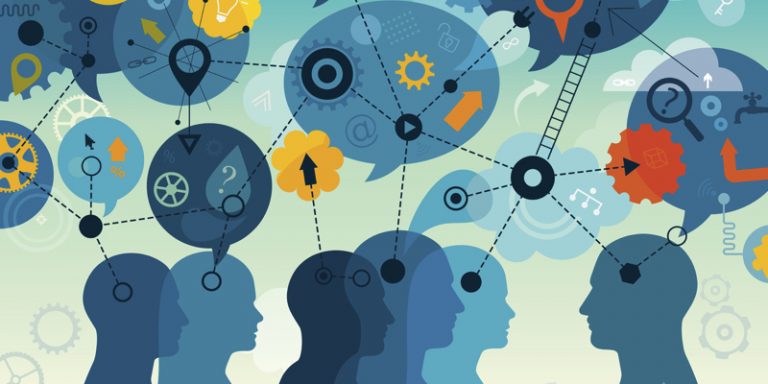
A three-year research project conducted by a UMons team as part of a project led by the start-up MoodMe has focused on analysing the “emotions” of participants. Artificial intelligence, which is already used to analyze images for the purpose of identifying people, is expected to be used to identify the emotions of Internet users, customers or spectators.
Two ways of identifying emotions
According to Matei Mancas, a researcher specializing in computational modeling of attention and founder of the Ittention spin-off from UMons, there are two modes of identifying and analyzing emotions:
- The interpretation, via algorithms, of the seven primary expressions (joy, sadness, surprise, anger, fear, disgust, contempt), which MoodMe uses in its solutions until now. A technique that requires a good quality of the databases on which the algorithms are trained. For this specialist, the major pitfall remains the predominance of white European or American faces in the image databases, which guide the algorithm:
“It has already been shown that some existing [algorithmic] models are bad or inefficient on black faces, for example. Because of the lack of reference faces. That’s why, at UMons, we are also working on an ethnicity model to eliminate bias.”
The other point of vigilance is the “veracity” of the image: is the emotion captured spontaneous, authentic or simulated? What about cultural bias? Are the seven primary emotions really universal? Matei Mancas points out that the techniques for identifying these emotions are based in part on the work of anthropologists, who guarantee serious conclusions.
In order to make the potential of emotion “calculation” usable on a smartphone, the UMons researchers aim to adapt neural networks, finding other means than the insufficient compression techniques. Neural networks that are light enough and yet efficient and fast enough to be installed in our mobile assistants.
- Another track, still based on algorithms, the FACS method (Facial Action Coding System), a method of describing facial movements developed in 1978 by psychologists Paul Ekman and Wallace Friesen. It is based on the study of facial muscles and those activated for each emotion, on a panel of emotions wider and more varied than the 7 primary ones. It allows a reading of emotions where several emotional states are combined (joy and fatigue for example). The relevance of the database is still important here, so it is necessary to check the conditions under which it was set up: quality of the images, exact description, seriousness of the authors, ethnic and/or cultural balance of the cohort…
Where to find these FACS baselines? Matei Mancas answers:
“It is more difficult to find FACS baselines. Some are usable [authorized as such by their authors] only for research purposes. The difficulty increases when you want to use them to launch a product, app or service on the market.”
The UMons project
For the purposes of the project, the UMons researchers wanted to have as many models as possible that specifically handle specific cases such as age, gender, ethnicity, etc. This multiplicity of models requires tenfold computing power and extensive tests to determine the interaction or cross-influences between several models with different themes. For example, in such and such a circumstance, for such and such an emotion, will a young person be more expressive than an older person? Is this model more effective on women or men, on young people or older people?
In order to continue the work, UMons must find new funding to advance this innovative research. Indeed, the track of embedded solutions (usable and operational in mobile mode or even on a connected object) is original. Matei Mancas believes that an embedded solution would be a valuable differentiating factor:
“No need to send images to the servers of a GAFA or a BATX.
Less risk of piracy, preservation of confidentiality, no dependence on platforms, etc., the consequences are numerous.
The ethical question
How far can we go with these FACS techniques? Should we define a framework for “virtuous” use? Could we take the step of predictive or even prescriptive use of FACS solutions? The types of potential applications are diverse and varied and often flirt with red lines: “guiding” a buyer according to the emotion he feels, allowing a company to anticipate his reactions and choices and adapt its proposals accordingly; optimising the behaviour or approach of salespeople or teamwork; advising an individual on how to make his presentations more effective during webinars; helping a teacher to better capture the attention of his students, etc.
Is emotion recognition a more sensitive subject than automated face recognition, which is already the subject of much debate? For Matei Mancas:
“If face recognition is questioned today, it is because it allows us to put a name on a face, to find an individual on a video, to “trace” him in the long term… On the side of emotion identification, we are talking more about a use that is not linked to a personal identification process. We aggregate and compare the data with a cohort, with other people.”
Despite this, he acknowledges the possibility of these techniques being used for nefarious purposes:
“It is therefore important to define a legal and ethical framework.”
How? By adopting, according to Matei Mancas, an “ethics by design” approach at the start of a project, i.e. training an algorithm and immediately deleting the data on the faces of the individuals on which the exercise is based, or by taking all possible guarantees to ensure that a hacker who takes control of a mobile application cannot get his hands on the data. There is a fine line between desirable and undesirable practices. This requires great vigilance in use and a supervised practice. A scheme similar to the tags that the RGPD defines for example could be implemented. A project has been launched at the UMons psychology faculty:
“Teaching teachers to recognize attitudes and reactions of their students, to fight against the loss of attention.”
With the guarantee that the data collected to create the analytical model will not be kept. And that no individual will be targeted.
Translated from Quand l’IA interprète les émotions humaines : focus sur le projet de recherche UMons – MoodMe