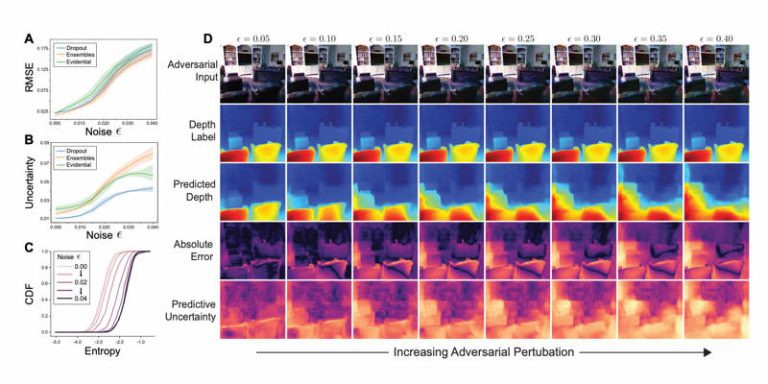
In a publication on arXiv, researchers from MIT and Harvard University presented Deep Evidential Regression, an artificial intelligence that would be “aware” of its own reliability and its own uncertainties and errors. Formed from neural networks, it proposes decisions and is also capable of measuring their uncertainty.
As researchers Alexander Amini, Wilko Schwarting and Ava Soleimany, Daniela Rus on arXiv indicate, deterministic neural networks are increasingly deployed in safety-critical areas where calibrated, robust and efficient uncertainty measures are crucial.
In their paper, they propose a new method for training non-Bayesian neural networks to estimate a continuous target and its associated evidence to learn both random and epistemic uncertainty. They achieve this by placing proof priors on the original Gaussian likelihood function and training the neural networks to infer the hyperparameters of the proof distribution.
They also imposed priors during learning so that the model is regularized when its predicted proofs are not aligned with the correct output. Their method does not rely on sampling during inference or on out of distribution examples (OOD) for algorithm training, thus allowing efficient and scalable learning of uncertainty. Researchers have demonstrated learning of well-calibrated measures of uncertainty at various benchmarks, scalability to complex computer vision tasks, and robustness to conflicting test samples and OODs.
Researchers have reported that they have successfully tested their artificial intelligence. To do this, as can be read in their publication, they presented her with a picture and she had to judge the depths in different parts of it. Their algorithm indicated when she was unable to judge with precision certain images that were outside her usual attributions.
Translated from Des chercheurs du MIT et de Harvard ont formé des réseaux de neurones capable d’estimer sa propre fiabilité