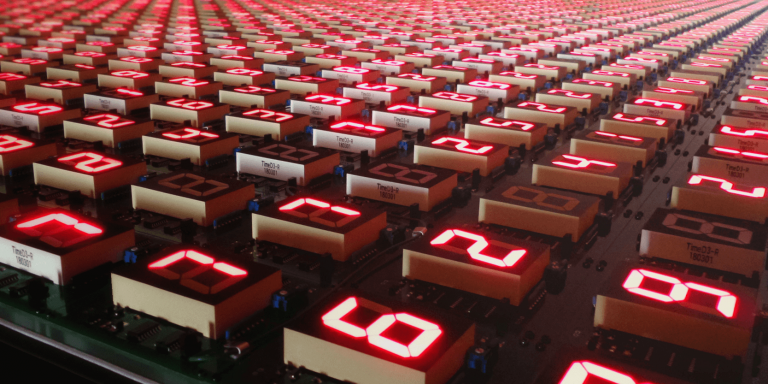
Mathematics plays a prominent role in the developments brought about by artificial intelligence in recent years: we think in particular of machine learning, or computational neuroscience. Recently, two researchers have started what could be a revolution thanks to posits, which are nothing more or less than a different way of representing numbers.
New cost calculations thanks to posits?
We have to consider a huge computing power to make revolutionary AI applications work. Do you know, for example, how many operations it took to train GPT-3, Open AI’s most advanced language model? Exactly one million billion billion. All at an estimated cost of $5 million.
But according to recent research in the field, cost reduction in artificial intelligence training is a possibility thanks to posits. Indeed, the two inventors of this approach, John Gustafson and Isaac Yonemoto, imagined their discovery as a new way of encoding real numbers. To understand their impact, one must keep in mind that this type of number cannot be perfectly encoded, because there are infinite numbers. In order to fit in a certain amount of bits (the smallest unit of information on a computer), real numbers must be rounded.
A major evolution for mathematics?
On some of them, especially the large positive and negative numbers, the precision of the encoding is increased. According to John Gustafson, “It’s a better match for the natural distribution of numbers in a calculation […] It’s the right precision, where you need it.”
The Complutense University in Madrid, for example, has developed a first processor core implementing the new posit standard. Their results were presented at the IEEE Symposium on Computer Arithmetic. The team that presented the results is composed of El-Mehdi El Arar, Devan Sohier, Pablo de Oliveira Castro, and Eric Petit.
Compared to the standard use of floating point numbers, it was found that the accuracy of a basic computational task is increased fourfold.
The training of large AIs at the heart of questions
A major mathematical revolution may therefore be underway. However, a practical implementation is necessary to prove the efficiency and the relevance of the posits in the training of large artificial intelligences. David Mallasen Quintana, a researcher at the Complutense University of Madrid, concludes: “[…] we now want to see how to train large artificial intelligences. People have tried them in software […] we now want to try them in hardware.”
The Madrid-based institution has already done performance comparisons between 32-bit floats and 32-bit posits. The results are encouraging, although these initial experiments suggest that the increase in precision does not affect computation time. Meanwhile, other research teams are working on their own hardware implementations to advance the use of posits.
Watch the video presentation of David Mallasén, Raul Murillo, Alberto A. Del Barrio, Guillermo Botella, Luis Piñuel and Manuel Prieto-Matias at the IEEE Symposium via this link
You can also access the presentation of John Gustafson, one of the two inventors of posits, at a seminar at Stanford University on the subject of next generation computer arithmetic.
Translated from Les posits, une révolution des mathématiques ?