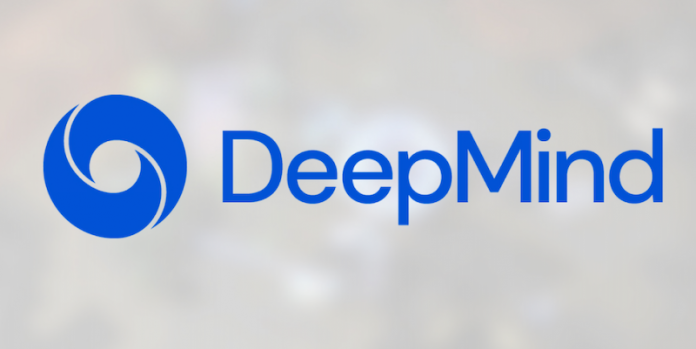
DeepMind, a subsidiary of Alphabet Group specialized in Artificial Intelligence, announced last December that it had designed a new language model based on Transformer: GOPHER, which, with more than 280 billion parameters, will allow it to test the limits of large natural processing models.
Language modeling enables the design of intelligent communication systems using large repositories of written human knowledge. DeepMind researchers conducted an analysis of Transformer-based language model performance across a wide range of model scales – from models with tens of millions of parameters to a 280 billion-parameter model called Gopher. Transformers were created to do translation, classification or text generation, but were soon exploited in many NLP (Natural Language Processing) tasks. They are able to adapt words according to the context.
GOPHER, a model with more than 280 billion parameters
To demonstrate that a more powerful language model is more efficient because of its size, DeepMind has developed this new model called GOPHER which contains 280 billion parameters, exceeding the 175 billion of Open AI’s GPT-3 but well below the 530 billion of Microsoft and Nvidia’s MT-NLG.
Gopher relies on a transformer, a deep learning model used by text generators like OpenAI’s GPT-3. Transformers are trained by huge networks pre-trained on massive amounts of unstructured text, capturing useful linguistic properties. The models are then refined and used in multiple cases: machine translation, text summarization, text capture… The attention mechanism allows them, unlike previously used recurrent neural networks, to process words independently of the order in which they were written, to process information differently and to adapt them according to the context.
As a result, DeepMind researchers have evaluated models of different sizes on 152 diverse tasks, achieving peak performance in the majority of cases. Scaling gains were greatest in areas such as reading comprehension, fact-checking, and identifying toxic language, but logical and mathematical reasoning were not convincing.
Environmental and Ethical Issues
On the one hand, very large language models are very energy intensive: they consume massive amounts of computing power and generate increasing amounts of carbon dioxide. On the other hand, the reproduction of bias in the generation of text strings produced by the models poses a real ethical problem. For DeepMind, “other challenges of AI text generation require, in their view, a solution that goes beyond data and computation. This includes producing stereotypical results or generating false content.” In these cases, DeepMind suggests adopting additional training routines, including feedback from human users.
Translated from Focus sur GOPHER, le nouveau modèle de langage naturel de DeepMind de plus de 280 milliard de paramètres