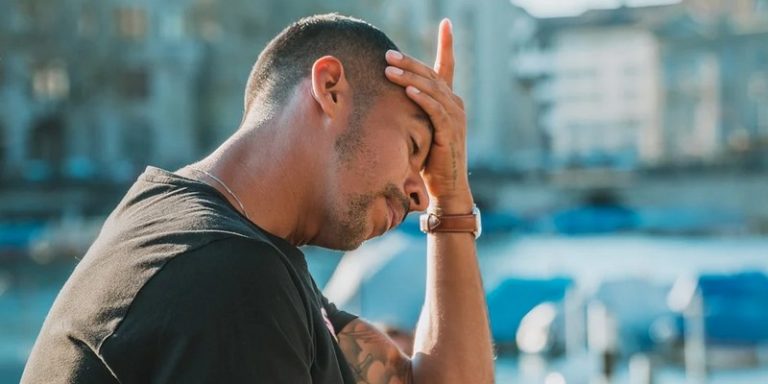
Among 15-24 year olds and students, suicide is the second leading cause of death. Based on this observation, a research team from INSERM and the University of Bordeaux used AI to try to understand this phenomenon. Thus, these researchers have identified four indicators that accurately predict the risk of suicide in young people and students. This study was conducted in collaboration with the University of Montreal and McGill University.
More than 5,000 students surveyed to predict suicide risk
“How to predict suicide risk among students? : this is the problem taken into account by the team of researchers from INSERM and the University of Bordeaux, in collaboration with the University of Montreal and McGill University, who have identified, thanks to AI, a restricted set of mental health indicators allowing to predict with precision suicidal behaviors of students and young people.
Christophe Tzourio, coordinator of the study, explains all the interest of this research:
“This work requires confirmation, but it opens up the possibility of large-scale screening by identifying, through short and simple questionnaires, students at risk of suicide in order to direct them towards appropriate care.”
The results of their study were the subject ofa scientificpublication written by Mélissa Macalli, Marie Navarro, Massimiliano Orri, Marie Tournier, Rodolphe Thiébaut, Sylvana M. Côté and Christophe Tzourio. Between 2013 and 2019, 5,066 French students were questioned to find these four indicators over a period of one year or more.
These students were over eighteen years old, French-speaking and enrolled in a French university. They had to fill in two detailed online questionnaires. The first one at the time of their enrolment and the second one at least one year later. Information on the participants’ health, drug and alcohol use, medical and psychiatric history, psychological state, etc. were collected. A great deal of data was collected in this way.
Thanks to this follow-up, the researchers were able to note that 17.1% of the participating students (17.4% of girls and 16.8% of boys) presented suicidal behaviours during the year that elapsed between the two questionnaires.
A machine learning method to classify predictive factors for suicidal risk
Using the questionnaires, the researchers identified 70 potential predictive factors that could influence suicidal behaviour. These include socio-demographic data, certain physical and mental health parameters, personal and family history of suicidal behaviour, living conditions and habits, substance use and childhood trauma.
The research team implemented a machine learning method that consists of simultaneously analyzing several factors associated with suicidal risk. From this analysis, a ranking of these 70 factors was drawn up, according to their importance in predicting suicidal behaviour in students.
The results of the study show that among the 70 potential predictors measured, four of them are able to detect about 80% of suicidal behaviors, they are:
- Suicidal thoughts,
- Anxiety,
- Symptoms of depression,
- Self-esteem.
With these results, the Rosenberg scale measuring self-esteem, Spielberger’s STAI-YB scale for anxiety and the PHQ-9 for depression, tend to be valid psychological scales that are sufficiently informative to identify students likely to exhibit suicidal behavior. Self-esteem is the most atypical marker of the four identified by the researchers, with Melissa Macalli, a doctoral student in epidemiology and lead author of the study, noting:
“The mental health specialists in our teams did not expect self-esteem to be one of the four major predictors of suicidal behaviour. This result, which would not have been obtained without the use of artificial intelligence techniques, which made it possible to cross-reference a large number of data simultaneously, opens up new perspectives in both research and prevention.”
Translated from Une équipe de recherche exploite le machine learning pour déterminer les indicateurs du risque suicidaire