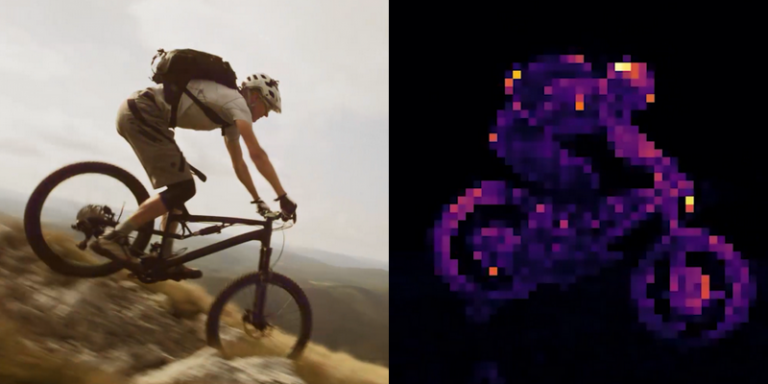
Facebook AI announcedat the end of last week two discoveries considered as major in artificial intelligence and computer vision. These models are the result of a collaboration with Inria and several academic institutions. The first, called DINO,is based on the transformation of vision through self-supervised learning, the second, PAWS, is a semi-supervised learning model that allows detailed results to be obtained with fewer learning steps than a more traditional model.
DINO, a high-performance self-supervised learning model for segmentation
DINO is a self-supervised learning model for vision transformation. This tool can analyze and segment objects, animals or plants present in the foreground of an image or a video thanks to an automated supervision. Below is an illustration explaining how this model works. On the left, a photograph of a dog, in the middle, the segmentation of the image generated by a supervised model and on the right, an example generated by the self-supervised model developed by Facebook AI.
In the following example, we can notice that DINO focuses on the animal in the foreground, although there may be a background that makes the segmentation more complicated:
This discovery was the subject of a paper published by Mathilde Caron, Hugo Touvron, Ishan Misra, Herve Jegou, Julien Mairal, Piotr Bojanowski and Armand Joulin for Facebook AI Research, Inria and Sorbonne University. Their research initially focused on self-supervised learning and computer vision and contributed to the creation of this new model.
Segmenting objects in an image is a complex operation that can be performed using computer vision. Its implementation allows to facilitate certain tasks such as the permutation of the background of an image with an object present in the first one or to allow robots to navigate on an image whose environment is cluttered. While usually a segmentation can be performed with a supervised learning model that requires a large amount of data, the design of DINO proves that self-supervised learning and an appropriate architecture can be very suitable.
Another finding concerns the right to use images. DINO-based models could potentially become copy or copyright infringement detection systems. Although this is not what the tool was designed for, the researchers noted that this task falls within the capabilities provided by the model.
PAWS, a method to get detailed results more efficiently
The other model developed by Facebook AI is called PAWS. It is a semi-supervised learning method that exploits a small amount of labeled images in order to obtain detailed results with 10 times less learning steps. These results were obtained when pre-training a standard ResNet-50 model with the PAWS method with only 1% of the ImageNet labels. The figure below shows the effectiveness of PAWS when pre-training with 1% of the ImageNet labels on the left and 10% of the labels on the right:
This feature was also the subject of a paper written by Mahmoud Assran, Mathilde Caron, Ishan Misra, Piotr Bojanowski, Armand Joulin, Nicolas Ballas and Michael Rabbat for Facebook AI Research, Inria Université Grenoble Alpes, Mila – Québec AI Institute and McGill University.
Unlike more traditional self-supervised methods that directly compare representations, PAWS uses a random subsample of labeled images to assign a pseudo label to unlabeled views. These pseudo-labels are obtained by comparing representations of unlabeled views with representations of labeled support samples. The model is then updated such that losses are minimal.
Below is a graph describing the operation of PAWS, with the anchored view in purple, the subsamples in green and the positive view in orange. From left to right, we can see the three steps: image acquisition, image representation, and pseudo labels:
This method is much less prone to superficial labeling adjustment unlike other semi-supervised learning models since it does not directly optimize the accuracy of the predictions they wish to give to labeled samples.
By combining DINO and PAWS, the AI research community will be able to create new computer vision systems that depend on less labeled data. The use of large amounts of unsupervised data provides an excellent opportunity to pre-train these transformation and segmentation models with a reduced image base.
Translated from Facebook AI annonce deux découvertes liées à la vision par ordinateur et à l’apprentissage supervisé