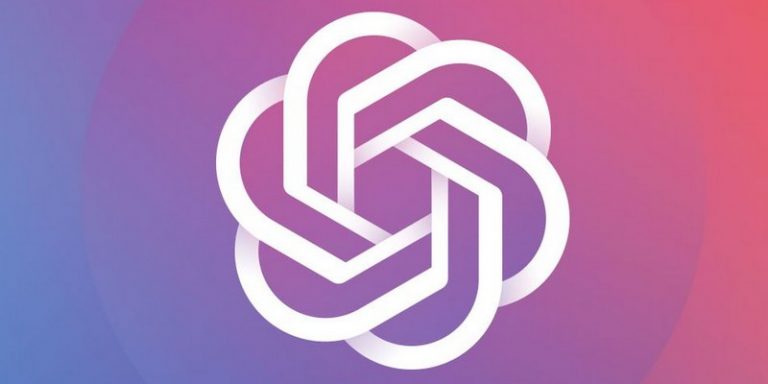
The experts at Open AI announced one of their latest findings around language model behavior. They noticed that they could improve it by fine tuning a small organized dataset with less than a hundred example values.
Fine Tuning
Large Generalist Models to Fit a Specific Context
Linguistic models can produce almost any type of text, in any type of tone or personality, depending on the user’s input. The approach proposed by the researchers aims at giving language model operators tools to reduce the set of behaviors of a model to a constrained set of values. Their process is called Process for Adapting Language Models to Society (PALMS).
Appropriate language model behaviour, in the same way as human behaviour, cannot be reduced to a single standard for all. These behaviors differ depending on the context or their application at a given time. The process developed by Open AI aims to take into account the context to improve the behavior by creating a value-targeted dataset.
This research was the subject ofan article by Irene Solaiman and Christy Dennison.
The three steps of the researchers’ proposed PALMS process
The method proposed by the researchers consists of three stages. It was developed while working on a use case for an API client in order to obtain a behavior that suited the research teams:
- First step: the experts selected categories deemed to have a direct impact on human well-being. These included abuse/violence/threat, physical and mental health, human characteristics and behaviours, injustice and inequality, all types of relationships (romantic, family, friends, etc.), sexual activity, and terrorism.
- Step two: The researchers developed a dataset of 80 values-focused text samples. Each sample was in the form of a question/answer between 40 and 340 words. This database weighs 120 kilobytes, or about 0.000000211% of the data used to train GPT-3. The GPT-3 models (containing between 125 million and 175 billion parameters) were refined on this dataset using tuning tools.
- Step 3: Three sets of models (GPT-3 Base Models, GPT-3 Models targeted to values that were refined on the previously discussed dataset, GPT-3 Models refined on a dataset of similar size and writing style) were evaluated using quantitative and qualitative measures such as human ratings to assess adherence to predetermined values, toxicity assessment, co-occurrence measures to examine gender, religion or origin.
Results of using this process
The research teams drew three samples per command line interface (CLI), with five CLIs per category for a total of 40 CLIs and 120 samples per model size. Three different humans also evaluated each of the samples. All were scored from 1 to 5, with 5 as the maximum score, corresponding to the text being fully associated with its sentiment. The results yield the following graph:
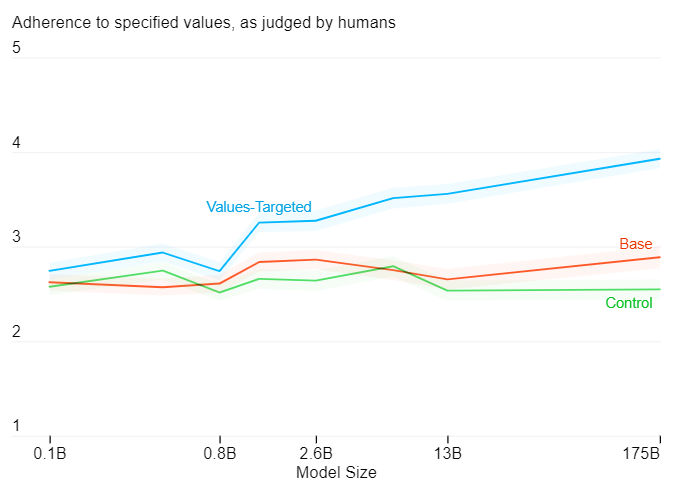
Human evaluations show that models focused on values from the dataset designed by the researchers appear to adhere more to the specified behavior. The more parameters the model has, the more effective the process appears to be. The project researchers state:
“Our analysis shows a statistically significant behavioural improvement without compromising performance on downstream tasks. It also shows that our process is more efficient with larger models, implying that people will be able to use relatively fewer samples to fit the behaviour of large language models to their own values. Given that defining values for large groups of people risks marginalizing minority voices, we sought to make our process relatively scalable compared to retraining from scratch.”
The research teams believe that language models need to be adapted to the society we are in and that it is important to take its diversity into account. They also add that in order to successfully design language models, AI researchers, community representatives, policy makers, social scientists, etc. can come together to understand together how the world wants to use these tools.
From a more practical point of view, this study allows OpenAI to reaffirm its vision of the relevance of a large generalist NLP model, sometimes accused of being too generalist to be really exploitable, by highlighting the possibility of adapting it to specific contexts.
Translated from Des chercheurs d’Open AI conçoivent un processus pour améliorer le comportement des modèles linguistiques