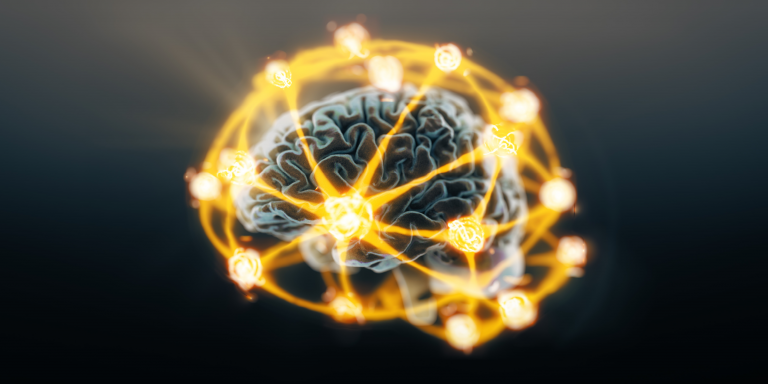
The potential of artificial intelligence to help overcome the challenges we face is certain, but the limited computational capabilities of conventional computers are a hindrance to solving certain problems. Quantum combined with AI could well be the solution. To demonstrate this, physicists from the University of Vienna, the National Research Council (NRC) and the Politecnico di Milano, led by Professor Philip Walther and Dr. Roberto Osellame, have designed a quantum optical memristor based on integrated photonics. Their study was published in the journal “nature photonics.”
Artificial neural networks, which simulate the biological structure of the brain, are the basis of artificial intelligence applications. Thanks to deep learning, they are gradually becoming autonomous, but to do so, they must be trained on large amounts of data. In addition to the volume, one of the biggest challenges of AI is the computing power needed to train neural networks.
With its combinatorial power, the quantum computer could reduce training times and processing times for many AI applications.
From classical memristor to quantum memristor
Described for the first time in 1971 by Leon Chua, the memristor (or memristance) is a passive electronic component, just like the capacitor or the resistor. This component, which consists of a ferroelectric layer enclosed by two electrodes, is of interest to researchers because of its ability to mimic the neurons and synapses of biological brains and its capacity for self-learning.
The first memristors were based on the transfer of ions or atoms in a material under the effect of an electric voltage and a sudden rise in temperature caused by the electric current, but consumed a lot of energy. This is why a research team involving CNRS, Thales and the University of Cambridge, developed in 2017, a new type of memristor based on a quantum property of matter, the tunneling effect, which allows electrons to jump through a very thin layer of electrically polarized ferroelectric material, like a magnet. Several other quantum memristor proposals were subsequently made, but all were faced with limited technological functionality.
The optical quantum memristor of the study
This new memristor is based on a laser-written integrated photonic circuit that is fully reconfigurable using integrated phase shifters, and is capable of producing memristive dynamics on single-photon states through a classical measurement and feedback scheme.
Thus, the researchers used single photons, or single quantum particles of light, that propagate along waveguides laser-etched on a glass substrate and are guided along a superposition of multiple paths. One of these is used to measure the flow of photons through the device and this quantity, through an electronic feedback scheme, modulates the transmission on the other output, thus obtaining the desired memristive behavior.
On the other hand, the researchers developed simulations showing that optical networks containing quantum memristors can be used to learn on both classical and quantum tasks, implying that the quantum memristor could be the missing link connecting artificial intelligence and quantum computing. Michele Spagnolo, lead author of the paper, concludes:
“Unleashing the full potential of quantum resources within artificial intelligence is one of the greatest challenges in current research in quantum physics and computer science.”
Philip Walther’s group, which co-led the study, also demonstrated that robots can learn faster when they use quantum resources and borrow patterns from quantum computing.
Article source: “Experimental photonic quantum memristor”
https://www.nature.com/articles/s41566-022-00973-5#citeas
Translated from Le memristor quantique, chaînon manquant reliant l’intelligence artificielle et l’informatique quantique ?