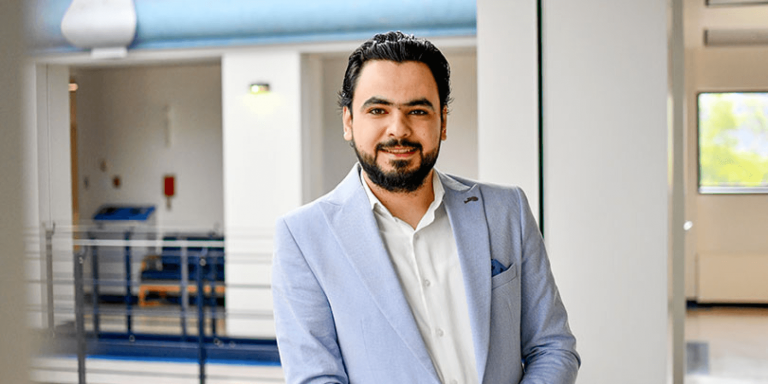
Heart rate variability is an indicator of heart health. Mohammad Moshawrab’s research on this topic received the best paper award at the 19th International Conference on Mobile Systems and Persuasive Computing (MobiSPC), held August 9-11 in Niagara Falls, Canada. Like the other papers accepted by MobiSPC 2022, ” Cardiovascular Events Prediction using Artificial Intelligence Models and Heart Rate Variability”is published by Elsevier Science in the online open access Procedia Computer Science series.
Mohammad Moshawrab is a doctoral student in engineering at the Université du Québec à Rimouski (UQAR), which welcomes about 6,700 students each year, including nearly 600 international students from more than 45 countries.
His doctorate in engineering aims to train specialists capable of designing and carrying out independently a research program to advance the state of knowledge in the engineering of physical systems and industrial processes.
His award-winning research, conducted with his thesis supervisor, computer science professor Mehdi Adda, and colleagues Abdenour Bouzouane of the Université du Québec à Chicoutimi, Hussein Ibrahim of the Institut technologique de maintenance industrielle, and Ali Raad of the Islamic University of Lebanon, focuses on the use of AI to predict cardiovascular disease.
Mohammad Moshawrab explains:
“We explain the technical process followed to preprocess the data and build four machine learning models. By comparing the results, we show that artificial intelligence is a promising avenue for predicting heart disease.”
AI to predict cardiovascular disease and save lives
Heart rate variability (HRV) is the variation in time between two heartbeats over a given period of time. Decreased HRV is associated with an increased risk of cardiovascular disease and may have predictive value for life expectancy and health.
In 2019, cardiovascular disease accounted for more than 32% of the global mortality rate. According to the WHO, the number of deaths from these diseases increased from 12.1 million in 1990 to 18.6 million in 2019.
HRV is also referred to as beat-to-beat variation in heart rate or RR peak interval duration, where R is a QRS complex wave extracted from a cardiac ECG signal.
The research team created and trained four models with “PhsyioNet Smart Health to assess event risk via ECG database” to analyze HRV characteristics and predict the occurrence of heart disease and cerebrovascular events.
Data from “PhsyioNet Smart Health to Assess Risk of Events via ECG Database.”
The data in this set were collected to measure the effectiveness of classifying hypertensive patients with higher risk of cardiac and cerebrovascular events characteristic of rate variability. It consists of 139 24-hour electrocardiographic (ECG) Holter recordings.
Each recording contains three ECG signals sampled at a rate of 128 samples per second with 8-bit precision.
The cohort consisted of 49 women and 90 men aged 55 years and older who were followed up for 12 months to record the occurrence of serious cardiovascular and cerebrovascular events such as: coronary revascularization, fatal and nonfatal acute coronary syndromes, syncopal events, myocardial infarction, fatal and nonfatal strokes, and transient ischemic attacks. During the follow-up period, 17 patients experienced a cardiovascular event, including 11 myocardial infarctions, 3 strokes, and 3 syncopal events.
Results of the study
The results obtained confirm the confidence in the use of AI in cardiology, with models based on support vector machines, deep neural networks, and XGBoost achieving 91.80%, 90.19%, and 89.10% accuracy, respectively.
Furthermore, adapting these models to work in real time will certainly help in implementing personalized and continuous monitoring to track the cardiac health of patients or people working in stressful environments or for very long periods of time.
Article source:
“Cardiovascular Events Prediction using Artificial Intelligence Models and Heart Rate Variability”.
https://doi.org/10.1016/j.procs.2022.07.030 Procedia Computer Science
- Mohamm Moshawrab, Department of Mathematics, Computer Science and Engineering, Université du Québec à Rimouski
- Mehdi Adda, Department of Mathematics, Computer Science and Engineering, Université du Québec à Rimouski
- Abdenour Bouzouane, Department of Computer Science and Mathematics, Université du Québec à Chicoutimi
- Hussein Ibrahim, Technological Institute of Industrial Maintenance, Quebec
- Ali Raad, Dean of the Faculty of Science and Arts, Islamic University of Lebanon
Translated from Prédire les maladies cardiovasculaires grâce à l’intelligence artificielle