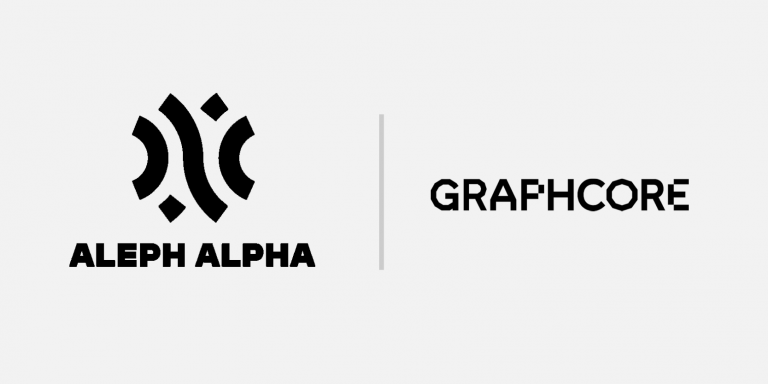
Last June, Graphcore and Aleph Alpha, two European AI leaders, announced that they would collaborate on the research and deployment of Aleph Alpha’s advanced multimodal models, including Luminous, on Graphcore’s IPU systems and the next-generation Good Computer. In mid-November, the partners unveiled the first fruit of their labors: the “Luminous Base” language model of 13 billion parameters sparse to just 2.6 billion parameters.
Graphcore, founded in 2016, is a British semiconductor company that develops gas pedals for AI and machine learning. The company has developed an “Intelligence Processing Unit,” or IPU, a microprocessor found on Graphcore’s IPU-POD datacenter computing systems.
This new type of processor has been specifically designed to meet the unique and specific needs required for AI and ML. It features granular parallelism, single precision arithmetic and sparsity support, among other features. Graphcore’s IPU systems accelerate AI and provide a competitive advantage in financial services, healthcare, consumer Internet, academic research and many other fields…
The IPU-POD 64, used for this benchmark architecture, maximizes available data center space and power, regardless of how it is provisioned. It can provide up to 16 petaFLOPS of AI computing for training and inference to develop and deploy on the same powerful system.
Aleph Alpha
Founded in 2019, based in Heidelberg, Germany, startup Aleph Alpha has been recognized by the Machine Learning, AI and Data Landscape (MAD) 2021 Technology Index as the only European AI company conducting generalizable artificial intelligence (GAI) research, development and design. It recently opened Alpha One, a commercial AI data center on the GovTech Campus Germany. Its ambition is to make the EU a major player in the field of AI and to consolidate its digital sovereignty.
Aleph Alpha develops novel multimodal models, including Luminous, combining computer vision with natural language processing (NLP) to process, analyze and produce a wide range of texts, presented at the International Supercomputing Conference (ISC) last year. The model is based on the company’s Multimodal Augmentation of Generative Models through Adapter-based Finetuning (MAGMA) method.
Jonas Andrulis, CEO and founder of Aleph Alpha, states:
“All of Europe’s linguistic and cultural diversity must be reflected in modern AI applications, as this is the only way for every European country, large or small, to benefit from the potential of new AI technologies. This ensures that the best of AI is not reserved for the few, but is available to all equally. With LUMINOUS and MAGMA, we are taking a big step in this direction. Moving forward, we want to work with partners to ensure that in Europe, we take our digital destiny into our own hands.”
The two partners presented a sparse variant of Aleph Alpha’s commercial chatbot Luminous at SC22 in Texas.
Luminous Base Sparse uses only 20% of the processing FLOPs and 44% of the memory of its dense counterpart. On the other hand, its 2.6 billion parameters can be fully retained in the ultra-fast on-chip memory of an IPU-POD Classic, optimizing performance.
Parameter reduction
Large language models such as OpenAI’s GPT-3 or deepMind’s Gopher rely on more than a hundred billion parameters, the next ones will have even more, requiring more and more computing power.
Most currently use dense models, where equal representation and computation is given to all parameters, whether or not they contribute to the model’s behavior. Aleph Alpha and Graphcore have removed the least relevant 80% of weights and re-trained the Luminous Base Sparse model using only the important weights. These are represented using the Compressed Sparse Row (CSR) format.
The computational FLOPS required for inference dropped to 20% of the dense model, while memory usage was reduced to 44%, as additional capacity is required to store the location and value information for the remaining non-zero parameters. In addition, the resulting model reduced the energy requirement by 38% compared to the dense model.
Selecting the important parameters
According to Graphcore and Aleph Alpha, parsimony is seen as an important counterbalance to the exponential growth in the size of AI models and the corresponding increase in computational demand.
Models for language, vision, and multimodal models require parameter scaling, increasing the costs of running them. Parameter selection would allow specialized submodels to run more efficiently and allow AI startups such as Aleph Alpha, to deploy highly efficient models with minimal computational requirements for customers.
Translated from Graphcore et Aleph Alpha présentent un modèle d’IA clairsemé à 80 %