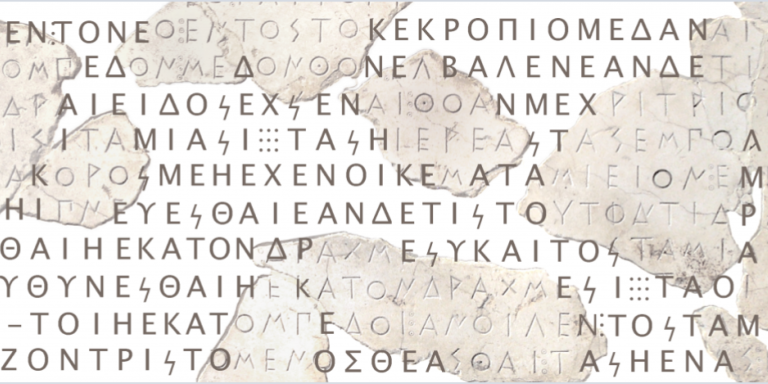
Epigraphy studies inscriptions on durable materials, such as stone and metal, and is very useful to historians for the study of ancient civilizations, most of whose perishable records have disappeared. However, these inscriptions on imputrescible materials have suffered the damage of time and many are damaged, illegible, sometimes pieces of their support are missing… The Deepmind team has developed Ithaca to help scientists restore these texts. It published its work in the journal Nature under the title “Restoring and assigning ancient texts using deep neural networks” in early March.
Deepmind is working to find the “best techniques from machine learning and systems neuroscience for powerful general-purpose learning algorithms.” This Alphabet company is particularly invested in rational and predictive reasoning, as well as deep learning and deep reinforcement learning methods. In 2019, Deepmind had developed with the University of Oxford, Pythia, an AI tool dedicated to epigraphy. The latter aimed to find the missing letters or text. Ithaca goes much further than Pythia as it allows to deduce the geographical origin and dating of inscriptions while carbon-14 dating cannot be applied to them.
This study was carried out in collaboration with the departments of Humanities of the Ca’Foscari University of Venice, the University of Oxford and the Department of Computer Science of the Economic University of Athens.
The study
Epigraphers must often reconstruct the missing text, a process known as text restoration, and establish the place and date of origin of the writing, tasks known respectively as geographical and chronological attribution. These three tasks are crucial steps in locating an inscription in history but are very complex, time-consuming, and specialized.
Deepmind’s team used its cutting-edge ML research to create Ithaca. Inspired by biological neural networks, CNNs can discover and exploit complex statistical patterns in vast amounts of data. Recent increases in computational power have enabled these models to meet challenges of increasing sophistication in many fields, including the study of ancient languages
Ithaca is a deep CNN architecture trained to simultaneously perform the tasks of textual restoration, geographic attribution, and chronological attribution, previously performed by epigraphers.
Ithaca (from Ithaca, the Ionian island of which Odysseus, the legendary hero, was king) was formed on inscriptions written in the ancient Greek language and throughout the ancient Mediterranean world between the 7th century BC and the 5th century AD. There are two reasons for this choice:
- the variability of the content and context of the Greek epigraphic record, which makes it an excellent challenge for language processing ;
- the availability of digitized corpora for ancient Greek, an essential resource for training machine learning models.
To train Ithaca in Greek inscriptions.
To train their model, the researchers created a pipeline to retrieve the unprocessed Packard Humanities Institute (PHI) dataset, which consists of the transcribed texts of 178,551 inscriptions and lists 84 ancient regions.
The resulting I.PHI dataset is, according to the team ,the largest machine-actionable multi-task dataset of epigraphic text, containing 78,608 inscriptions.
The results
While Ithaca alone achieves 62% accuracy when restoring damaged texts, once historians use Ithaca their performance jumps from 25% to 72%, confirming the impact of this synergistic research aid.
Ithaca can link inscriptions to their original discovery site with 71% accuracy and date them within about 27 years of the “inferior” dating proposed by modern re-evaluations. Ithaca’s predictions are, on average, only 5 years away from the newly proposed field truths.
Collaborating with historians
Ithaca can assist in the restoration and attribution of newly discovered or uncertain inscriptions. The team has created a publicly accessible open-source interface: https: //ithaca.deepmind.com, allowing historians to use Ithaca for their own research, while facilitating its development for other applications? The methods introduced in this research can be applied to any discipline dealing with ancient text (papyrology, numismatics, codicology), to any language (ancient or modern), also integrating additional metadata (inscription images, stylometry). The team concludes:
“Furthermore, the essentially interactive nature of Ithaca as a collaborative search aid lends itself to an effective setup for future machine learning research by adding humans into the training loop.”
Article Sources:
Ithaca was designed and researched by Yannis Assael*, Thea Sommerschield*, Brendan Shillingford, Mahyar Bordbar, John Pavlopoulos, Marita Chatzipanagiotou, Ion Androutsopoulos, Jonathan Prag and Nando de Freitas. Assael, Y., Sommerschield, T., Shillingford, B. et al. Restoring and attributing ancient texts using deep neural networks. Nature 603, 280-283 (2022). https://doi.org/10.1038/s41586-022-04448-z.
Translated from DeepMind présente Ithaca, Deep Neural Network pour la restauration et l’attribution de textes anciens