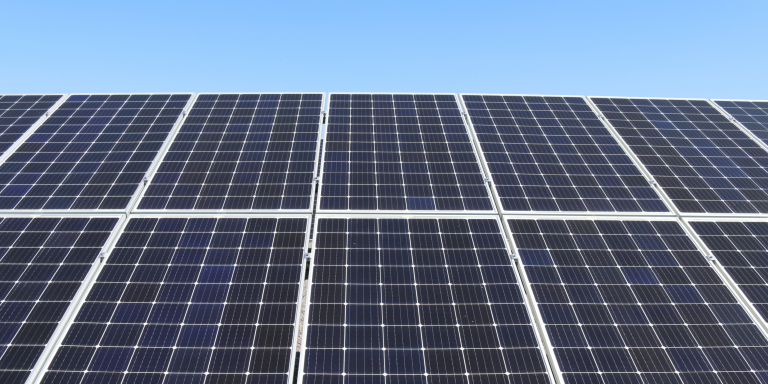
Hugo Le Picard, researcher at the Ifri’s Energy & Climate Center, and Mathieu Toulemont, Senior Machine Learning Engineer at PhotoRoom, sought to measure the progression of decentralized solar power in urban areas in Africa. To do so, they used deep learning methods to analyze 2.4 million satellite images of 14 sub-Saharan cities. They published their study entitled “Decentralized Solar in African Cities. An original analysis of satellite analysis and Deep Learning” in the Ifri Briefings on January 18.
The French Institute of International Relations (Ifri) was founded by Thierry de Montbrial in 1979, he is in fact its President and states:
“The
French Institute of International Relations (Ifri) is the leading French institution for research and debate on international relations. It is also a unique platform for understanding the contemporary world. In 40 years, it has become a reference on a global scale, recognized by its peers.
Hugo le Picard works in particular on issues of energy poverty, access to electricity and financing of electrical infrastructure in sub-Saharan Africa. He conducted this study, which showed that decentralized solar systems were increasingly present in cities that are covered by the electricity grid, with Matthieu Toulemont.
An unreliable electricity grid
In 2016, the World Bank stated:
”
Currently, only one in three Africans has access to electricity, with residents often condemned to rely on kerosene or spend hours in the dark. The region’s power companies are cash-strapped, burdened by aging infrastructure and unable to provide reliable power to their customers. If nothing is done to remedy this situation, there will be more Africans without electricity in 2030 than today.”
The Covid-19 crisis has caused the number of people without access to electricity to rise again despite real electrification efforts.
The major obstacle for those connected is the unreliability of the grid. There are frequent power outages and even if the grid is working, voltage drops limit the use of electrical appliances. Nigeria, for example, has a grid connection rate of about 83.9 percent in urban areas, but the grid only works 50 percent of the time. This weakness of the sub-Saharan power grids also has a considerable negative effect on economies, representing on average, depending on the country, a cost ranging from 1 to 5 percent of national gross domestic product (GDP).
The African continent is experiencing rapid demographic growth: the UN predicts that the sub-Saharan population will double by 2050 to reach 2.1 billion people.
The increasing need for electricity due to economic and demographic growth, its high price and the unreliability of the networks have encouraged consumers to self-sufficiency for their electrical consumption through diesel generators or decentralized solar systems, even in urban areas.
Satellite image analysis using Deep Learning and spatial econometrics
The researchers limited their analysis to fourteen cities. They used Google’s Maps Static API to collect satellite images of square areas with a radius of 2.5 kilometers (km) around geolocated points in the Afrobarometer socio-economic database. More than 2.4 million images, representing a total area of approximately 4.6 billion square meters, were downloaded.
To analyze the satellite images, a pipeline built around two different CNN models was established to first determine the geolocation of the solar panels and then extract the area. First, a classification model gave the probability of a panel being present in an image. Then, a segmentation model was applied to determine the area of the panel.
The data collected from satellite imagery does not allow the model to differentiate between residential solar panels on the one hand and solar panels installed on commercial facilities on the other. To overcome this problem, the team used a machine learning method: a Gaussian Mixture Model (GMM).
Conclusions of the study
The analysis of millions of satellite images in 14 cities in sub-Saharan Africa showed that the installed decentralized solar capacity in these cities is between 184 MW and 231 MW, which already represents almost 10% of the installed solar capacity in the region (excluding South Africa). Extending this analysis to other African cities would therefore most likely increase this estimate significantly.
Econometric analysis using the Afrobarometer socio-economic data cross-tabulated with the collected data found that those in the wealthier classes are most likely to adopt decentralized solar systems, regardless of the reliability of their power supply from the centralized grid.
Sub-Saharan utilities face a critical problem: it is the creditworthy customers who are turning to hybrid solutions.
Article sources: Hugo Le Picard and Matthieu Toulemont, “Decentralized solar power in African cities. An original analysis of satellite analysis and Deep Learning” Ifri Briefings, Ifri, 18 January 2022.
ISBN: 979-10-373-0463-6
Translated from Afrique : un chercheur de l’IFRI étudie le déploiement des panneaux solaires en zone urbaine grâce au deep learning