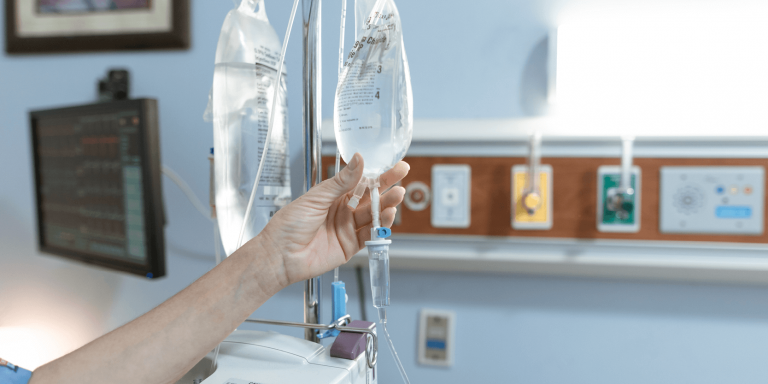
Intensive care units (ICUs) provide continuity of care and continuous monitoring for critically ill patients. A team of researchers from Chiba University Graduate School of Medicine conducted research to investigate the predictive accuracy of mortality and length of stay of patients admitted to ICUs using machine learning. Their study, “Prediction algorithm for ICU mortality and length of stay using machine learning,” was published in Nature on July 28.
Shinya Iwase, Taka-aki Nakada, Tadanaga Shimada, Takehiko Oami, Takashi Shimazui, Nozomi Takahashi, Jun Yamabe, Yasuo Yamao, Eiryo Kawakami from Japan’s Chiba University Graduate School of Medicine, are the authors of this study. The objective was to investigate the predictive accuracy of mortality and length of stay of patients admitted to the ICU but also to identify variables contributing to the accurate prediction or classification of patients. Most existing studies have focused only on mortality.
The Study Data
The investigators conducted this retrospective cohort study using electronic health record data from the admission of 12,747 patients to the ICU at Chiba University Hospital, Japan, from November 2010 to March 2019.
To develop the prediction algorithms, data for 91 input variables were collected, as soon as possible after admission and no later than 24 hours, from the ICU data system.
These variables included:
- Patient characteristics (age, gender, height, weight, blood type, clinical service categories, admission diagnosis, admission route: from emergency room, general ward, operating room, other hospitals) and comorbidities (acquired immunodeficiency syndrome, acute myeloid leukemia/multiple myeloma, heart failure, lymphoma, respiratory failure, cancer metastases, liver failure/cirrhosis, immunocompromised status, and dialysis);
- Blood tests (complete blood count, biochemistry, coagulation and blood gas analysis);
- Physiological measurements (HR, blood pressure, respiratory rate, peripheral oxygen saturation and body temperature).
Numerical data with an entry rate of less than 50% were not used for prediction.
Using the entry variables from the training cohort, the area under the curve (AUC) was analyzed in the test cohort to assess the predictive accuracy of the supervised machine learning classifiers, including random forest (RF) for outcomes (primary endpoint was mortality, secondary endpoint was length of ICU stay). Classification of variables that contributed to machine learning prediction was confirmed, and cluster analysis of patients with risk factors for mortality was performed to identify important variables associated with patient outcomes.
Machine learning using random forest revealed a high predictive value for mortality, with an AUC of 0.945 (95% confidence interval 0.922-0.977). In addition, RF showed high predictive value for short and long ICU stays, with AUCs of 0.881 (95% CI 0.876-0.908) and 0.889 (95% CI 0.849-0.936), respectively.
Lactate dehydrogenase (LDH) was identified as a contributing variable to accurate prediction in machine learning for mortality and ICU length of stay but also as a contributing variable to classification of patients into subpopulations based on different mortality risk factors.
The study’s conclusions
For the researchers, their study has several limitations. Because it was a single-center retrospective study, the accuracy of the prediction should be validated in a future prospective study. On the other hand, inconsistent timing of sample collection from patients could potentially affect the accuracy of predictions. In addition, the prediction of length of stay in intensive care was conditional on survival. Finally, prediction was performed on the basis of data collected at a single point in time of admission.
Real-time prediction may be useful for improving the accuracy of predicting ICU mortality and length of stay for critically ill patients whose condition is subject to abrupt changes. Future investigations using sequential data are warranted.
Nevertheless, this study demonstrated that the machine learning algorithm could predict ICU mortality and short/long ICU stay with high accuracy. Furthermore, LDH was found to be a key variable predicting both mortality and length of stay, and contributed to the grouping of ICU patients based on mortality risk.
Article Sources:
“Prediction algorithm for ICU mortality and length of stay using machine learning,” Nature, 07/28/2022. https://www.nature.com/articles/s41598-022-17091-5
Authors: Shinya Iwase, Taka-aki Nakada, Tadanaga Shimada, Takehiko Oami, Takashi Shimazui, Nozomi Takahashi, Jun Yamabe, Yasuo Yamao, Eiryo Kawakami.
Translated from Prédire la mortalité et la durée du séjour en unité de soins intensifs grâce au machine learning