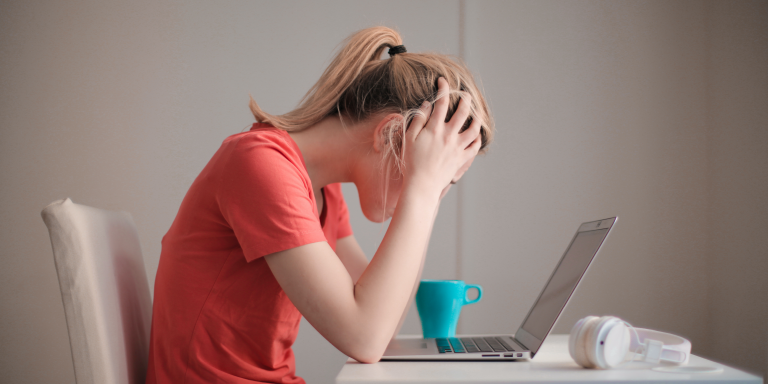
Burnout is a state of deep physical and psychological fatigue resulting from chronic stress in the workplace. Mascha Kurpicz-Briki, professor of data engineering at the Bern University of Applied Sciences in Biel, and her team of scientists supported by the Swiss National Science Foundation (SNSF) have just developed a method for detecting burnout, based on automatic text analysis. The study entitled ” BurnoutEnsemble: Augmented intelligence to detect indications of burnout in clinical psychology” in “Frontiers in Big Data.
In a study of nearly 10,000 working adults from 8 European countries, it was found that 18% of those surveyed felt stressed on a daily basis, and 30% of them to such an extent that they were considering changing jobs. This stress can lead to burn-out.
The clinical identification of this syndrome is difficult because the symptoms resemble those of depression or anxiety, among others. To detect it, psychologists rely on psychological tests in which patients fill out a questionnaire by checking off multiple-choice questions such as the “Maslach Burnout Inventory”, composed of 22 multiple-choice questions divided into three categories: burnout, depersonalization/loss of empathy and personal performance evaluation.
Example of a typical question-answer: “I feel burned out at the end of my work day: never/sometimes/every day”. Some people do not dare to check the answers “never” and “every day” or are tempted to lie to influence the results.
More comprehensive questionnaires with open-ended questions can also be used to detect burnout. Although they provide more relevant information, they require a lot of analysis and are therefore not applied.
Research based on text analysis
Mascha Kurpicz-Briki’s team has used artificial intelligence in a method that automatically analyzes texts and identifies, on this basis, whether the language is burn-out or not. The goal is to study whether these free-text questions are effective and to develop an automated metric to evaluate these questions. With success: the method correctly identifies 93% of burn-out cases. The scientist states:
“Automatic language processing is effective in detecting burnout while being time-efficient, which is very promising.”
Data collection
For this work, the scientist and her team analyzed texts from the Reddit platform, an English-language community website that functions as a discussion forum organized by topic. Although there is a subreddit dedicated to burnout, the number of entries was too small to provide a sufficiently large data set, so the team added texts from various thematic forums.
This resulted in a dataset of 13,568 samples describing first-hand experiences, 352 of which were related to burnout and 979 to depression. She then used machine learning to develop a method that assesses whether a text falls under burnout or not.
Specifically, she first classified the collected text excerpts:
- Text from burnout threads was manually categorized to exclude text where burnout referred to something else.
- Text from other, non-mental health related threads was labeled as not related to burnout.
Based on these examples, she trained several models. Each model used different configurations to determine whether a text (never seen by the model) contained indications of burnout or not. These models were then pooled in the diagnostic method, which proved to be very effective.
While these results are promising, the collaboration of medical experts is needed in the next step to verify the conclusions of this study on real cases of burn-out and on a representative sample of the population. The data collected on Reddit are indeed anonymous.
The authors emphasize that this work is oriented towards the augmented intelligence approach rather than artificial intelligence: instead of replacing clinical professionals, it strives to adopt a technology that empowers humans in the decision-making process, providing information to be taken into account in human decision making.
Article sources: G. Merhbene, S. Nath, A. Puttick, M. Kurpicz-Briki: Burn-outEnsemble: Augmented Intelligence to Detect Indications for Burn-out in Clinical Psychology. Frontiers in Big Data (2022). https://doi.org/10.3389/fdata.2022.863100
Translated from Intelligence artificielle : Détecter le burn-out grâce au traitement naturel du langage (NLP)