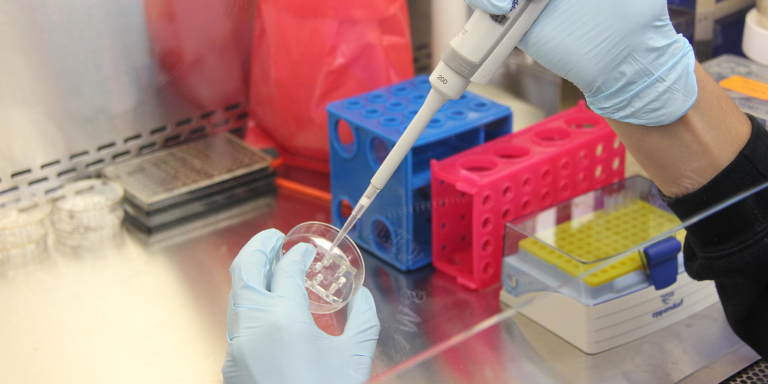
Researchers at the Singapore-MIT Alliance for Research and Technology (SMART) in Singapore, have developed an anomaly detection model that uses machine learningto identify the presence of possible microbial contamination within minutes.
The MIT Research Enterprise in Singapore has developed a new method for detecting microbial contamination in mesenchymal stromal cell (stem cell) cultures. It enables rapid and accurate testing of cell therapy products for use in patients. This method is a rapid alternative to conventional sterility testing and other microbiological bacteria detection methods, which often take several days and are almost always performed on finished products.
In the context of biotherapies, cell therapy aims to treat an organ or an organism by providing cells, mostly obtained from stem cells, to replace or support failing cells. In recent years, cell therapy has become an indispensable solution for treating a wide range of diseases, injuries and pathologies. In addition, it is showing increasing promise in treating certain cancers, autoimmune diseases, spinal cord injuries and neurological disorders. For this reason, Singaporean researchers at the Interdisciplinary Research Group (IRG) of the Critical Analysis for Personalized Medicine Manufacturing (CAMP) of the Singapore-MIT Alliance for Research and Technology (SMART) continue to refine cell culture manufacturing methods and processes to ensure the safety, efficacy and sterility of these products. By using ML to predict whether a culture is clean or contaminated, researchers save time and resources, as contaminated cultures can be quickly discarded and immediately reconstituted as Yie Hou Lee, SMART CAMP’s scientific director, explains:
“Our enhanced adoption of machine learning in microbial anomaly detection has enabled us to develop a unique test that quickly performs contamination control, marking a huge step forward in simplifying the manufacturing process for cell therapies. In addition to ensuring the safety and sterility of cellular products prior to injection into patients, this method also provides cost and resource efficiency for manufacturers, as it allows batches to be decisively stopped and restarted in the event of culture contamination.”
Translated from Ce modèle de machine learning détecte les contaminations microbiennes dans les cultures cellulaires