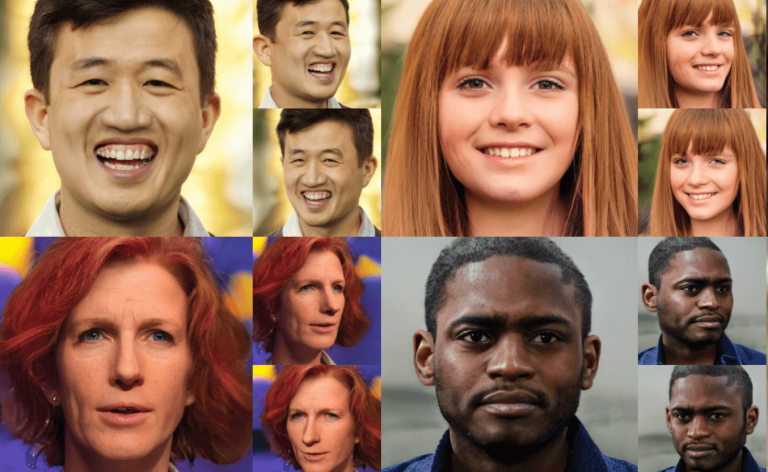
Transforming 2D images into 3D scenes is the subject of much research, Nvidia Research recently presented Instant NeRf, an AI model capable of doing this very quickly, various software are offered for free for this purpose on the Internet. Researchers at Stanford University and NVIDIA have used GANs (Generative Antagonist Networks) to create realistic 3D renderings. Their study titled “Efficient Geometry-aware 3D Generative Adversarial Networks” has been published on Arxiv and shared on the Github platform.
Unsupervised generation of high-quality 3D images using only collections of single-view 2D photographs has long been a challenge. Existing 3D GANs are either computationally intensive or make approximations that are inconsistent in 3D, limiting the quality and resolution of the generated images.
In this study, Stanford and Nvidia researchers improved the computational efficiency and image quality of 3D GANs without relying too heavily on these approximations. Training a GAN with neural rendering is expensive, they chose to introduce a hybrid explicit-implicit expressive network architecture that, in combination with other design choices, not only synthesizes coherent multi-view high-resolution images in real time, but also produces high-quality 3D geometry.
This representation combines an explicit backbone, which produces features aligned in three orthogonal planes, with a small implicit decoder. Compared to a typical multilayer perceptron representation, it is more than seven times faster and uses less than one-sixteenth more memory.
By decoupling feature generation and neural rendering, their framework can take advantage of state-of-the-art 2D CNN generators, such as StyleGAN2, and inherit their efficiency and expressiveness.
Results of the study
Although the resulting shapes show significant improvements over those generated by previous 3D-compatible GANs, they may still contain artifacts and miss finer details, such as teeth and require some improvements.
However, by combining an efficient explicit-implicit neural representation with an expressive pose-sensitive convolutional generator and a dual discriminator, this approach could enable significant advances towards 3D-supported photorealistic image synthesis and high-quality unsupervised shape generation.
This can enable rapid prototyping of 3D models, more controllable image synthesis and new techniques for shape reconstruction from temporal data.
Article source:
Efficient Geometry-aware 3D Generative Adversarial Networks ArXiv:2112.07945v2
AUTHORS:
Eric R. Chan, Stanford University, NVIDIA,
Connor Z. Lin, Stanford University
Matthew A. Chan, Stanford University
Koki Nagano, NVIDIA
Boxiao Pan, Stanford University
Shalini De Mello, NVIDIA
Orazio Gallo, NVIDIA
Leonidas Guibas, Stanford University
Jonathan Tremblay, NVIDIA
Sameh Khamis, NVIDIA
Tero Karras, NVIDIA
Gordon Wetzstein, Stanford University