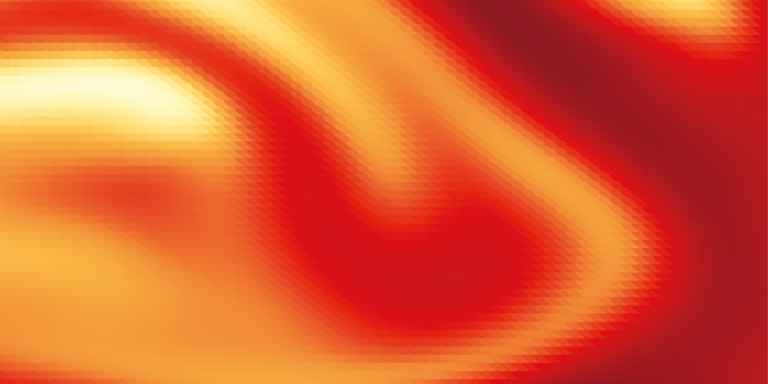
One of the most studied aspects of magnetic confinement fusion is the turbulence of the edge plasma, which is critical to the performance and operation of the reactor. Abhilas Mathews, a doctoral student in the Department of Nuclear Science and Engineering and works in the Plasma Science and Fusion Center located at MIT, has undertaken research on edge plasma phenomena to better understand the mechanisms that influence turbulence and stability in fusion plasmas.
Nuclear fusion is a natural phenomenon: the sun converts astronomical amounts of hydrogen into helium, producing a significant amount of energy. Researchers are working to reproduce this phenomenon and demonstrate that fusion of hydrogen atoms is possible. China and the United States seem to have made serious technological progress in this field and in France, the international ITER project is also advancing rapidly.
To design and develop magnetic confinement fusion energy reactors, researchers must understand the turbulent motion of plasmas: a mixture of ions and electrons swirling in reactor vessels. Plasma particles, following magnetic field lines in toroidal chambers called tokamaks, must be confined long enough for fusion devices to produce significant net energy gains, a challenge when the hot edge of the plasma (more than a million degrees Celsius) is only a few centimeters away from the much cooler solid walls of the vessel.
A new approach based on Deep Learning
Abhilash Mathews, a doctoral student in the Department of Nuclear Science and Engineering working at MIT’s Plasma Science and Fusion Center (PSFC), has focused his research on the plasma edge. This turbulent boundary is critical to understanding plasma confinement, plasma feeding, and potentially damaging heat fluxes that can strike material surfaces-factors that impact the design of fusion reactors. To understand and predict plasma behavior, researchers use numerical simulations, complex and time-consuming calculations. “Scaled-down” computer models that run much faster, but with significant levels of accuracy, help speed up Braginski’s research.
For decades, scientists have used Braginski’s reduced two-fluid theory to simulate plasmas despite uncertain accuracy. Abhilash Mathews combines physics and machine learning, he explains:
“A successful theory is supposed to predict what you are going to observe, for example, temperature, density, electric potential, fluxes. And it’s the relationships between these variables that basically define a theory of turbulence. What our work is essentially looking at is the dynamic relationship between two of these variables: the turbulent electric field and the electron pressure.”
After demonstrating through deep learning a way to calculate the turbulent electric field from a fluctuation in electron pressure in the plasma consistent with reduced fluid theory, Abhilash Mathew investigates this connection in more detail, comparing it to higher fidelity turbulence simulations. He then finds that, in plasmas relevant to existing fusion devices, the predicted turbulent fields of the reduced-fluid model are consistent with the high-fidelity calculations. In this sense, the reduced turbulence theory works. But to fully validate it,“you have to check every connection between every variable,” Mathews explains.
Mathews’ advisor, principal investigator Jerry Hughes, states:
“This work shows that, under the right set of conditions, physics-based machine learning techniques can paint a very complete picture of the rapidly fluctuating edge plasma from a limited set of observations. I’m excited to see how we can apply this to new experiments, in which we almost never observe all the quantities we want.”
These physics-based deep learning methods open new avenues for testing old theories and expanding what can be observed from new experiments. David Hatch, a researcher at the Institute for Fusion Studies at the University of Texas at Austin, believes these applications are the beginning of a promising new technique. He states:
“Abhi’s work is a major achievement with broad application potential.For example, given limited diagnostic measurements of a specific amount of plasma, physics-informed machine learning could infer additional amounts of plasma in a nearby domain, thereby increasing the information provided by a given diagnosis. The technique also opens up new strategies for model validation.”
Abhilas Mathews concludes:
“Translating these techniques into fusion experiments for real on-board plasmas is a goal we have in mind, and work is currently underway, But this is just the beginning.”
Translated from MIT : Etudier le mouvement turbulent des plasmas grâce à l’IA