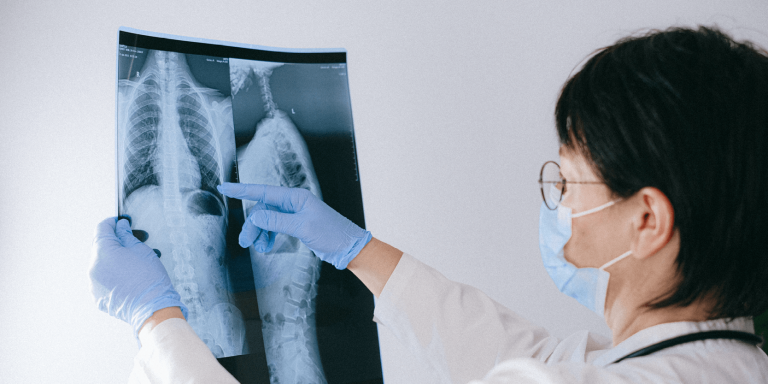
Tuberculosis is a contagious disease caused by the bacterium Mycobacterium tuberculosis. It affects the lungs, but can also affect other parts of the body such as the brain or kidneys. To detect it, the WHO recommends a chest X-ray. However, low-income countries where this disease is most common lack experts to interpret it, researchers have developed a deep learning system to detect active pulmonary tuberculosis on chest X-rays and compared its performance to that of radiologists. The study, ” Deep Learning Detection of Active Pulmonary Tuberculosis at Chest Radiography Matched the Clinical Performance of Radiologists,” was published in the journal Radiology.
Radiology is a journal published by the Radiological Society of North America, RSNA, an association of radiologists, radiation oncologists and medical physicists.
Tuberculosis kills more than one million people worldwide each year, and the COVID-19 pandemic has exacerbated the problem. One in four people worldwide is infected with the bacillus Mycobacterium tuberculosis, and 5% to 10% of these people will develop active tuberculosis (TB) in their lifetime. About 90% of active infections occur in about 30 countries, most of which have limited resources to combat this public health problem.
Using AI to detect lung disease
Studies based on deep learning to screen chest x-rays followed by confirmatory nucleic acid amplification testing (NAAT) have shown better cost-effectiveness than using NAAT alone.
WHO evaluated 3 computer-assisted detection systems and determined that their accuracy and diagnostic performance were similar to that of human readers. Because of the shortage of experienced readers, WHO now recommends computer-assisted detection for screening and triage in persons aged 15 years or older.
The Study
For this retrospective study, researchers developed a deep learning system (DLS) to interpret chest radiographs for imaging features of active pulmonary TB. They tested it on datasets from China, India, the United States, Zambia, and South Africa and evaluated it under two conditions:
- When it had a single predefined operating point on all data sets;
- When it was customized based on the radiologist’s performance in each region.
Because diagnostic performance can be influenced by disease prevalence, they compared DLS with two different groups of radiologists: one based in a TB-endemic region (India) and the other in a nonendemic region (United States).
Next, they estimated the cost savings of using this DLS as a triage solution for NAAT in screening settings. This study aimed to model real-world deployment scenarios and assess generalizability in four areas with high TB rates and limited resources.
The DSL was trained on 165 754 images from 22 284 individuals. For the 1st round of testing, the data came from China, India, the United States, and Zambia, the test set consisted of 1236 images, 212 of which were identified as positive for TB based on microbiological or NAAT tests. These were scored binary by 9 radiologists from India and five from the United States.
Results of the study
The DLS achieved a higher sensitivity than the 9 Indian radiologists’ analysis, at 88% versus 75%, with a non-inferior specificity of 79% versus 84%. The performance of the DLS was otherwise excellent in two case-control data sets commonly used in China and the United States.
The second set of tests involved subjects from a gold mining population in South Africa, a group with a high prevalence of tuberculosis, but also lung problems such as silicosis, emphysema and chronic obstructive pulmonary disease. DLS performed similarly to radiologists, as it did for HIV patients, but in this series, performance was much poorer.
Study co-author Sahar Kazemzadeh, a software engineer at Google Health, states:
“What is particularly promising about this study is that we looked at a range of different datasets that reflected the breadth of TB presentation, different equipment, and different clinical workflows .”
The AI system met the thresholds set by WHO in 2014 for any TB test in most datasets, according to Bram van Ginneken, professor of medical image analysis at Radboud University Medical Center in Nijmegen, the Netherlands, a co-author of the study. It could be very useful for low-income countries because the modeling could lower screening costs by 40 to 80 percent.
For Sahar Kazemzadeh:
“Filling the expert shortage is where AI comes in,. We can teach computers to recognize TB from X-rays so that, in these low-resource environments, a patient’s X-ray can be interpreted in seconds. “
Article sources: ” Deep Learning Detection of Active Pulmonary Tuberculosis at Chest Radiography Matched the Clinical Performance of Radiologists “
Radiology, DOI:10.1148/radiol.212213
Authors:
Sahar Kazemzadeh, Jin Yu, Shahar Jamshy, Rory Pilgrim, BEng, Zaid Nabulsi, Christina Chen, Neeral Beladia, Charles Lau,Scott Mayer McKinney, Thad Hughes, Atilla P. Kiraly, Sreenivasa Raju Kalidindi, Monde Muyoyeta, Jameson Malemela, Ting Shih, Greg S. Corrado, Lily Peng, MD, Katherine Chou, Po-Hsuan Cameron Chen, Yun Liu, Krish Eswaran, Daniel Tse, Shravya Shetty, Shruthi Prabhakara.
Google Health, 1600 Amphitheatre Pkwy, Mountain View, CA 94043 (S.K., J.Y., S.J., R.P., Z.N., C.C., N.B., S.M.M., T.H., A.P.K., G.S.C., L.P., K.C., P.H.C., Y.L., K.E., D.T, S.P.); Advanced Clinic, Deerfield, Ill (C.L.); Apollo Radiology International, Hyderabad, India (S.R.K.); Department of Tuberculosis, Center for Infec-tious Disease Research in Zambia, Lusaka, Zambia (M.M.); Sibanye Stillwater, Weltevreden Park, Roodepoort, South Africa (J.M.); and Clickmedix, Gaithersburg, Md (T.S.).
Translated from Le deep learning pourrait permettre de détecter la tuberculose plus précocement dans les pays à faibles revenus